The financial landscape has undergone a significant transformation in recent years, driven by technological advancements and changes in consumer behavior. Credit cards have become an integral part of modern commerce, offering convenience and flexibility in payment options. However, this widespread use of credit cards has also given rise to new challenges, primarily related to fraud detection and credit risk assessment. In this era of big data, financial institutions are leveraging advanced analytics, machine learning, and data management techniques to address these challenges effectively.
The literature in this field is vast and dynamic, reflecting the ongoing evolution of data analytics and financial technology. This paper aims to provide a structured overview of the current state of credit card analytics, drawing insights from a wide range of scholarly articles, research papers, and industry reports. This study explores the challenges faced by financial institutions, discusses the existing solutions and best approaches, and presents recommendations for future research directions. Our objective is to equip readers with a comprehensive understanding of the intricacies of credit card analytics, enabling them to make informed decisions and contribute to advancements in this critical domain.
Credit risk assessment is a pivotal component of the financial sector, determining the likelihood of a borrower defaulting on their credit obligations. With the surge in credit card usage, assessing the creditworthiness of individuals has become paramount for financial institutions.
Traditional Credit Scoring • Traditionally, credit scoring was based on a borrower’s credit history, income level, employment status, and other static factors. Financial institutions would use these factors to determine the creditworthiness of an individual. However, these methods often lacked the granularity and adaptability to account for the dynamic nature of an individual’s financial behavior.
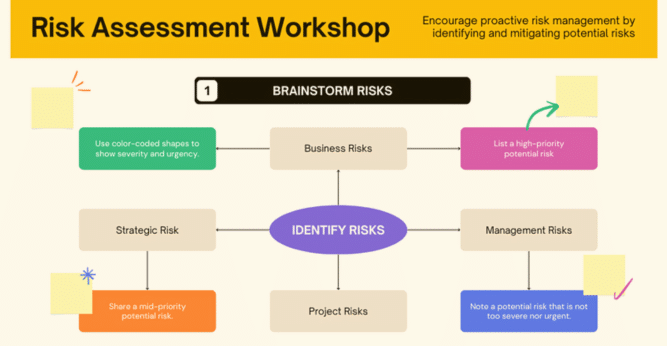
Photo Courtesy: Suresh Dodda
Evolution to Machine Learning • Integrating machine learning into credit risk assessment has brought about a paradigm shift. Machine learning models, trained on vast datasets, can predict an individual’s likelihood of default with greater accuracy. By analyzing vast amounts of transactional data, financial institutions can gain deeper insights into an individual’s spending habits, payment patterns, and financial behavior. This data-driven approach allows for a more nuanced and accurate assessment of credit risk.
Big Data and Credit Risk. The advent of big data technologies has further enhanced the capabilities of credit risk assessment models. These technologies enable the processing and analysis of vast datasets in real-time, allowing for dynamic risk assessment.
The future of credit risk assessment lies in integrating advanced technologies like artificial intelligence, blockchain, and quantum computing. There is also a growing emphasis on ethical AI, ensuring that credit risk assessment models are transparent, fair, and free from bias.
Furthermore, integrating alternative data sources, such as social media activity, online behavior, and even biometric data, can provide a more holistic view of an individual’s creditworthiness.
Credit risk assessment has evolved from static, rule-based models to dynamic, data-driven systems. Integrating machine learning, big data technologies, and advanced analytics has enhanced the accuracy and efficiency of credit risk models. As technology advances, so will the capabilities of credit risk assessment models, ensuring that financial institutions can lend with greater confidence and reduced risk.
The credit card analytics domain presents various challenges that organizations must navigate to succeed in fraud detection, risk assessment, and data analytics. These challenges encompass multiple aspects, from data security to technological scalability. Data Security and Privacy Data security and privacy are paramount concerns in credit card analytics. As financial institutions handle vast amounts of customer data, ensuring its security and privacy is of utmost importance. Breaches can lead to significant financial losses and damage an institution’s reputation, eroding customer trust]. Compliance with data protection regulations, such as the General Data Protection Regulation (GDPR) in Europe and the Health Insurance Portability and Accountability Act (HIPAA) in the United States, is essential.
Achieving a balance between data security and usability is a persistent challenge in the industry, as stricter security measures may impact the accessibility and usability of data for analytics purposes.
Data Quality and Integrity Maintaining the quality and integrity of data is fundamental to credit card analytics. Flawed or inaccurate data can lead to erroneous insights and decisions, potentially resulting in significant financial losses. Data quality encompasses various dimensions, including accuracy, completeness, timeliness, consistency, uniqueness, validity, and traceability. Assessing and ensuring data quality throughout its lifecycle, from acquisition to processing, is an ongoing challenge. Implementing data quality frameworks and data governance practices is critical to address this challenge.
Solutions and Best Approaches Addressing the challenges in credit card analytics requires a proactive approach and adopting best practices. Financial institutions can implement various solutions and strategies to enhance data security, quality, compliance, and analytics capabilities.
Advanced Encryption Techniques To mitigate data security concerns, financial institutions can employ advanced encryption techniques. These techniques ensure that sensitive data, even if breached, remains unintelligible to unauthorized entities. By encrypting data both at rest and in transit, organizations add an extra layer of protection to customer information. Advanced encryption standards like AES.
About Author
Suresh’s technical prowess extends to AI/ML, where he has contributed to research papers. His effective management skills have consistently ensured timely project delivery within allocated budgets. His extensive international experience includes working with esteemed clients such as Dubai Telecom in Abu Dhabi, Nokia in Canada, Epson in Japan, Wipro Technologies in India, Mastercard in the USA, National Grid in the USA, Yash Technologies in the USA, and ADP in the USA.
Within core industries such as banking, telecom, retail, utilities, and payroll, Suresh possesses a deep understanding of domain-specific challenges, bolstered by his track record as a technical lead and manager for globally dispersed teams. Suresh’s professional stature is further underscored by his membership in prestigious organizations like IEEE. His contribution as a journal reviewer for IGI Global and Judge for technology innovation awards like GLOBEE highlights his knowledge and expertise in technology and research.
Published by: Holy Minoza